Using ML models to predict the occurrence of invasive species based on habitat preferences
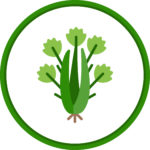
Invasive species are posing significant ecological, socio-economic, and human health threats to our society. It is imperative to accurately predict the potential occurrences of invasive species in order for management to concentrate efforts on prevention, early detection, and swift response. This study aims to employ various ML algorithms to forecast the likelihood of invasive species occurrences based on habitat preferences. We compared the prediction accuracies of three ML algorithms: Random Forest, Logistic Regression, and Gaussian Naive Bayes. Our analysis utilized data collected from twelve lakes located in the Adirondack region of Upstate New York. The outcomes of our study reveal that the Gaussian Naive Bayes model exhibited markedly higher accuracy levels compared to both the random forest and the logistic regression model. These findings highlight the effectiveness of the Gaussian NB model in predicting invasive species occurrences, underscoring its potential as a valuable tool for proactive management and conservation efforts.